Feb. 15, 1988: Neural networks generate interest and innovation in artificial intelligence
Advances in computing technology and data storage made neural networks more promising than ever, sparking widespread investment into the technology.
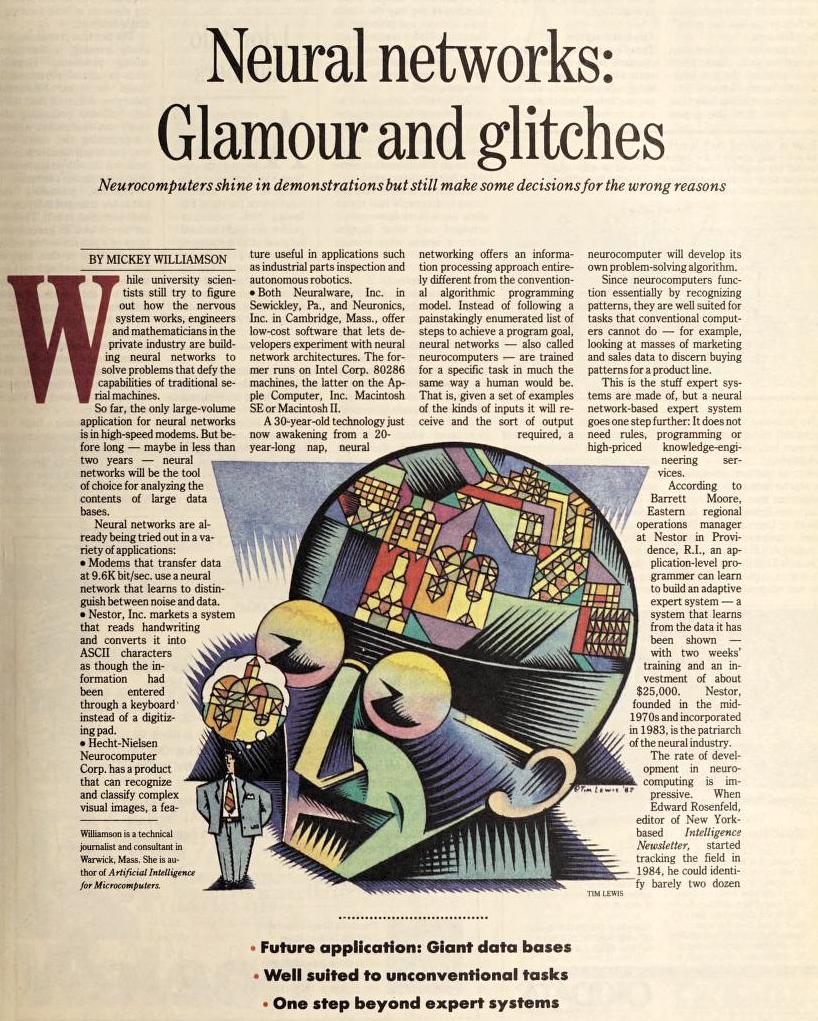
In recent years, academic researchers and tech industry leaders have invested heavily in neural networks, a type of algorithm used in artificial intelligence. Although today artificial intelligence is hugely popular and was estimated to have generated over $60 billion in 2020, it has undergone several “summers” and “winters” over the last few decades as its popularity repeatedly spiked and fell. In 1988, an article in Computerworld magazine discussing the “glamour and glitches” of neural networks was published at the tail end of an AI summer during which hundreds of new companies were founded using neural network technology.
Although AI development has been transformed in recent years with the emergence of powerful computing resources, the mathematical foundations of neural networks were already established in the 1980s. Computerworld magazine defined the concept: “neural networking offers an information processing approach entirely different from the conventional algorithmic programming model,” avoiding a specific list of steps in favor of being “trained for a specific task much in the same way a human would be.” Though today many comparisons between the mechanisms of learning in artificial intelligence and the human brain are largely superficial, the origins of the field drew directly from what was known about the brain. Neuroscientists hoped their research to understand biological learning processes would allow for better computer models of learning.
An industry leader told Computerworld, “Neural networks will be the tool of choice for analyzing the contents of large data bases.” This prediction has become reality today, with large data and neural networks going hand-in-hand to generate artificial intelligence products spanning countless applications. At the time of the article, exciting products included one that could “recognize and classify complex visual images, a feature useful in applications such as industrial parts inspection and autonomous robotics.” Image recognition products have become a cornerstone of AI applications.
Progress in artificial intelligence research was limited by the types of computational resources available to researchers in the 1980s. Researchers stated that “some problems involve such extensive amounts of data, or the patterns to be matched are so complex, that special-purpose hardware will have to be developed.” This proved to be true: with every advance in computing hardware, AI development accelerated even more. Researchers were cautious to apply their neural networks since the networks may “pick out bogus variables” in its decision-making. The complexity of the networks also meant that it these systems could not “explain their decisions the way more conventional rule-based systems [could].” Similar concerns exist today about modern AI systems, which have been shown to make faulty decisions and often lack of interpretability and explainability.