Modeling movement: understanding internally displaced migration
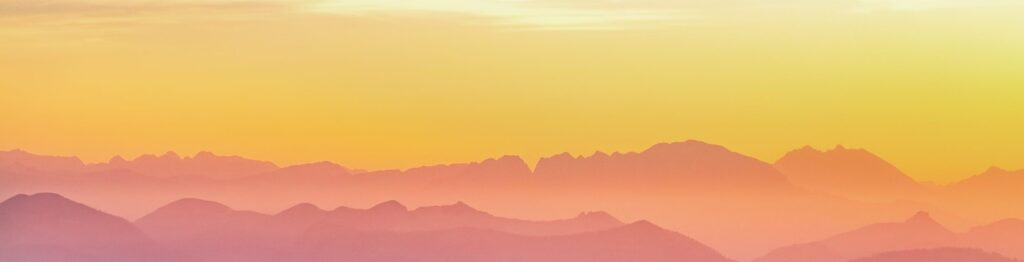
Editor’s Note: This article originally appeared on the Cloudera Foundation website. In April 2021, the Cloudera Foundation merged with the Patrick J. McGovern Foundation.
by Ethan Harrison
June 23, 2020
How data could help target and expedite humanitarian resources to IDPs
By the end of 2019, 45.7 million people were internally displaced by conflict and violence. Almost half of these internally-displaced persons (IDPs) are under 14 years old or over 60. Struggling to survive in the world’s most fragile and conflict-affected states, IDPs risk being left behind in the United Nation’s 2030 Agenda for Sustainable Development.
Humanitarians are working daily to respond to forced displacement and help meet IDPs’ basic needs for survival. However, there are clear practical challenges: IDPs are on the move and living in hard-to-reach areas. This is where better data and evidence can make a difference. It can provide a foundation for understanding the dynamics of displacement – who is going where and why – and improve the ability of humanitarians to target their work to reach these populations. If humanitarian actors know where and how IDPs move, they can better target their assistance to reach these populations before it is too late.
More needs to be done…to generate reliable data on IDPs’ location and demographics and the patterns and duration of their displacement. Without such information, neither governments nor humanitarian and development stakeholders are able to plan effective interventions to reduce the impacts of displacement or the risk of it happening in the future.
– IDMC Global Report on International Displacement 2020
Our solution
Our approach models the routes that IDPs take after they are displaced. Understanding these migration routes – and destination – in real-time can help humanitarians target their aid to reach people in need. Once disaster strikes and IDPs are forced to move, their top priority is to reach their destination as quickly and safely as possible. What they regard as safe and viable is shaped by a variety of factors, such as topography, availability of infrastructure and services, and the intensity of violence in surrounding regions. In other words, IDPs are rational actors: They base their migration routes on factors that they can observe, and that we can model.
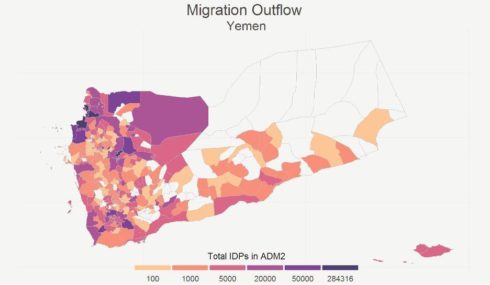
We pilot this framework using data on IDPs’ migration within Yemen, collected by the International Organisation for Migration’s (IOM) Displacement Tracking Matrix. To understand how different factors shape IDPs’ migration patterns, we use spatially referenced data at the district level in Yemen on the location of conflict events, the topography of the region, the density of the population, the level of economic activity, and other factors relevant to IDPs’ decision of where and how to migrate. With simple machine learning models, we are able to accurately predict the size of migration inflow ~80% of the time.
All of this points to the fact that we can forecast IDP migration in real-time with this approach. Based on our modelling approach, we built an interactive, web-based platform that allows decision-makers to forecast potential migration flows. Users can click on a district in Yemen where data is available and simulate migration to nearby districts.
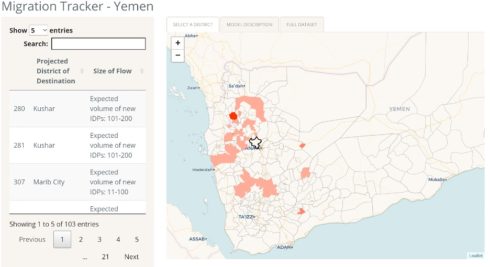
Next steps
Being able to now-cast IDPs’ migration patterns has the potential to dramatically improve humanitarian responses to forced displacement. So, what’s next? We want to scale this research to advance the frontiers of research on the dynamics of displacement. Here are clear, actionable ways we can do so, building on methods highlighted by the IDMC in their latest forced displacement report:
- Mobile technology: Locational services in mobile phones have the potential to unlock novel approaches to modelling forced displacement. Prior attempts to predict migration due to violent conflict have almost always focused on stocks of inward and outward flows. By using locational services data, we will be able to build models that are not limited by the same constraints of time or space, allowing us to examine previously unobserved factors like a migrant’s return to their origin, length of displacement, and exact flight route. These datasets can complement official sources to provide unique insight into forced displacement and its determinants.
It’s important to note that the mobile tech revolution has occurred at a pace such that privacy, data security, and compliance measures are not always in place. Some countries have yet to adopt any sort of reliable data protection standards for mobile users, leaving the burden of security on the researcher. Once more, the potential harmful implications of big data on a given mobile user are just being understood. The use of these datasets for IDPs comes with obvious ethical concerns especially given the potential to expose actual locations of IDPs. Our approach to personal privacy consists of producing only aggregate results and anonymizing specific phone numbers for analysis. Most importantly, we plan to only share results once they no longer pose a threat to expose pockets of internally displaced persons that could further endanger these populations. - Fieldwork: Interviews and on-the-ground data collection can help improve the quantitative models from our research and allow us to gain deeper insight into why and how IDPs move. Participatory research (IDMC, 2020) with formerly displaced people can help inform gaps in knowledge about forced displacement and its dynamics.
We plan to integrate these two approaches to build a capable tool that can inform humanitarian aid allocation in conflicts from an early onset. Together, the two approaches could be an answer to a major constraint in our response to a growing phenomenon while advancing the collaborative use of spatial analytics and field research.
Sources: Global Report on Internal Displacement (GRID). (2020). Internal Displacement Monitoring Centre (IDMC).https://www.internal-displacement.org/sites/default/files/publications/documents/2020-IDMC-GRID.pdf
Ethan Harrison is a recent graduate of the College of William and Mary with degrees in data science and economics.